An article by Ligence team for Life Sciences Baltic conference 2021
The hype is real
There is so much hype on the AI in the social media that we thought there must be some clarification to it. We will help you explain why any new medical technology must be taken cautiously.
First, AI will not replace physicians or nurses, or anyone and it won’t because it is not as intelligent as you may think. In many cases AI fails to perform in the real clinical setting, it makes mistakes and gives very small value to its end-users. Take for instance Google’s attempt to scan for diabetic retinopathy. They introduced a Deep Learning solution to hospitals in Thailand where the specialist to patient ratio is very low. The demand for specialist-level diagnostic measures was mountain high, therefore 11 clinics signed up for the pilot version of the new solution. Nurses only needed to produce pictures of patients’ eyes and the AI will do the rest, they said. The Deep Learning model worked really well in a lab setting, however it failed miserably when it was deployed in the real world. It actually created even more burden and frustration for medical specialists than it was before.
Second, the AI does not give so much value that it could replace anyone. When we got into the AI-in-healthcare business we met many great physicians who had already put their hands on several AI tools in healthcare. Most of these were used in radiology departments (makes sense because everything is digital there). We spoke to many radiologists and their opinion on any AI helping them find a nodule in lungs or brain is as helpful as having a medical student sitting behind them and telling them they see a nodule. It takes only several milliseconds for a radiologist to spot it and thus AI, despite looking cool, actually does not give anything valuable. Moreover, it fails in several more ways. It mistakes a nodule for a mammary gland (student level mistake, honestly) in AP chest radiographs. It detects nodules and presents them to a radiologist even though a radiologist cannot see one. Imagine the level of frustration it gives a medical professional who spent years training simply for an AI to tell one that he or she is mistaken.
In brief, the above problems make AI solutions not so attractive after all but is it?
Finding the real value
Even though it seems that AI is a bit too much overhyped, it can give value nevertheless. One just has to look for it.
First, identify pain points in a healthcare facility. For it to happen, one must actually speak to professionals working there. It is insufficient to be a top-notch data scientist and launch a startup that claims to solve every possible problem in healthcare. That’s why medical doctors are imperative to a healthcare startup company.
Second, any solution you propose must be usable by medical professionals. Have in mind that they are an extremely busy type and most likely will not take any novelty in a positive manner. Any innovative solution must be an easy-to-use product so that no one has to lose a minute figuring out how to do it. Therefore, one must thoroughly think about the implementation of their product.
In brief, medical doctors and other medical professionals are the only ones who know real problems in the clinical setting and they are the ones who should be asked how to solve them and how to implement solutions.
Good examples
AI is superior in mining and analysing large sets of data. There are companies that analyse invoices from medical institutions and then give feedback if unnecessary hospitalisations or other mistakes were made. There is a registry in the United States that allows dermatology specialists to compare their clinical behaviour to other specialists in his or her country based on a large amount of clinical data received nationwide. The benefits of such systems allow medical professionals and institutions to hold high standards thus improving healthcare in general.
The advantage of natural language processing has already been taken. Having a medical image viewing and reporting system with built-in language processing is becoming a necessity in modern radiology. A good example here can be Lifetrack Medical Systems, a company, that has implemented natural language processing into their reporting systems so that a radiologist can simply speak out loud his or her impression and it will automatically land to the radiology report. This allows for radiology examinations to become faster than before.
One of the most promising solutions in modern medicine that we have seen is in the drug discovery industry. There are companies making the drug discovery process several times quicker than before all thanks to smart algorithms capable of generating new drug molecules. This cuts down a decade from the time and millions from a budget required to create a new molecule before launching it to clinical trials.
In summary, AI application in healthcare can range a lot – from smart image reporting systems to data processing and performance reviews. It is a duty of a healthcare innovator to find out where can AI be applied.
Our experience
We started our project when we identified a process that has never been fully automated before – 2D echocardiography, an examination usually carried in outpatient clinic to evaluate the heart’s function and structure. This examination involves so much button pressing that it actually may take the majority of the examination itself. The pain was clear – too much manual work. The solution was to use Deep Learning algorithms that will be trained to recognise different heart pictures and perform different measurements on them so that a medical professional can skip doing them manually. Now, the implementation. We were hesitant if we should do it for the real-time examination or we install it for post-processing? Luckily, our team is comprised of medical doctors thus we have good connections in healthcare and eventually we decided to stick with the post-processing. Other medical doctors, especially cardiologists, expressed their opinion about our tool. Their opinion was uniform. They were happy to finally get a solution that allows them to skip the most unpleasant and time-consuming work and leave it for a machine to do it. They were not sceptical about it because the solution does not interfere with their superior opinion but simply gives measurements that can later be edited by a medical practitioner. We continue implementing our solution into healthcare institutions by integrating it with current systems operating within. We find it to be the most comfortable fashion for our end-users.
In brief, we at Ligence believe that our solution will definitely reshape the cardiology department and have a positive impact on cardiovascular health in our societies.
AI in the pandemic
The SARS-CoV-2 proved two things. The current healthcare and disease prevention systems are not really that effective in the face of a pandemic and, therefore, we need smart systems installed in healthcare settings as soon as possible. Smart systems are the ones who can predict a new pandemic before it even starts or help physicians to fight it more effectively when it has already started. A Canadian startup – BlueDot, has been actually nine days ahead of WHO when spotting a novel disease outbreak. Its algorithms indicated that unusually large numbers of atypical pneumonias had been lately emerging in one of Chinese cities what later become known as COVID-19. Furthermore, AI can even help learn more things about a new disease faster. A newly deployed solution by qure.ai is capable of Natural Language Processing and it reads X-rays. This allows the system to read a statement written by a physician when sending a patient for a radiological examination and then correlate findings in an X-ray image with symptoms presented in that statement. The more data we get and the more data is structured to make sense – the better the understanding of this novel disease is.
Our company chose a different approach to help fight the pandemic. More and more evidence has been appearing that the SARS-CoV-2 infection also causes cardiovascular manifestation especially in elderly patients for example Takotsubo cardiomyopathy, myocarditis and others. The method of choice in these situations remains heart ultrasound, therefore we figured we can apply our knowledge and newly discovered findings to come up with a solution helping physicians to diagnose the most common cardiovascular implications in COVID-19 positive patients. Since then we are developing a software capable of recognising those most common cardiovascular pathology findings in heart ultrasound images. We believe this software will decrease the average examination time and help physicians find the correct diagnosis quickly and more accurately anywhere in the world regardless of their previous heart ultrasound experience.
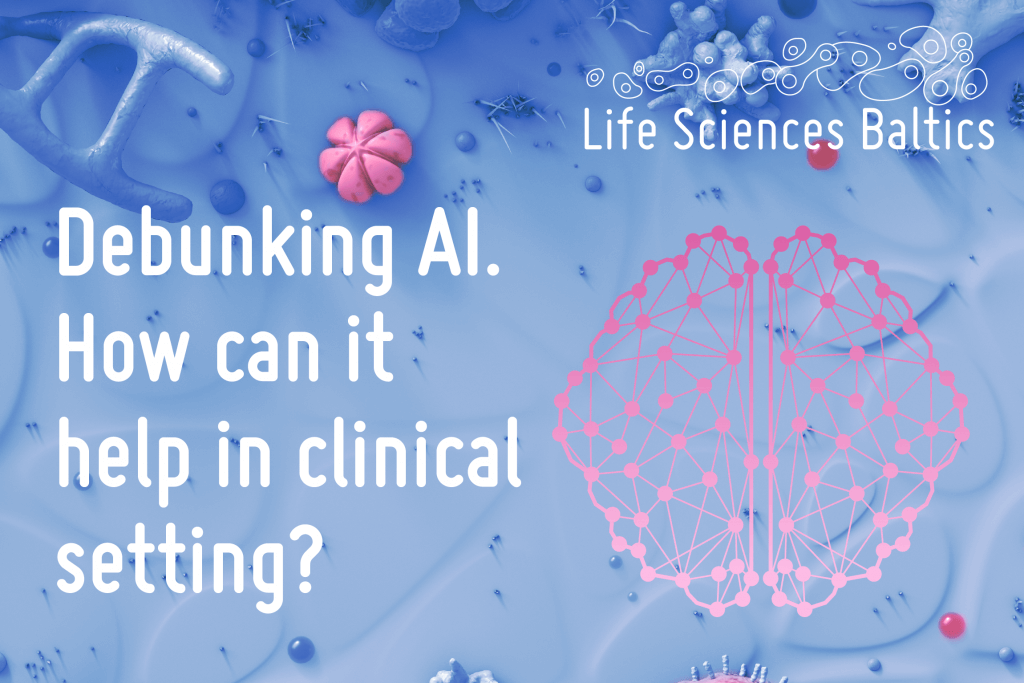